Aaditya
Ura
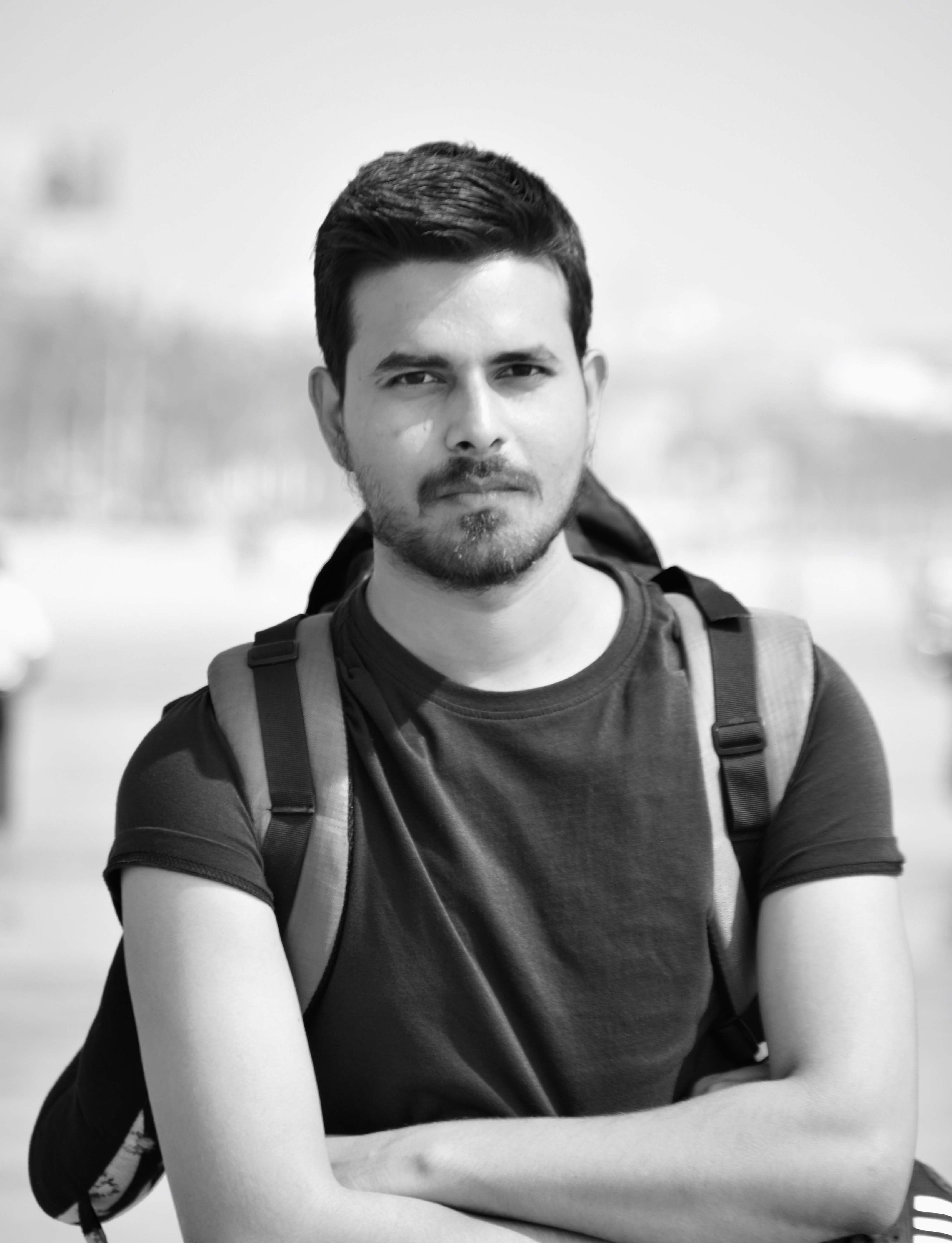
aadityaura@gmail.com
Hi there, I’m Aaditya (Ankit), a Senior Research Engineer specializing in NLP, Deep learning, and Machine learning. My research aims to develop machine learning methods in the Healthcare domain.
I currently work at Saama, conducting research to accelerate clinical trials and reduce drug development timelines. Before joining Saama, I worked as an NLP and Backend Engineer at Prescience. My research interests involve Representation Learning on Graphs, Federated learning, XAI, Generative language models and their applications in Healthcare data.
I’ve been honored to contribute meaningful research and datasets that have been adopted by leading companies like Facebook AI (Galactica), Google AI (Med-PaLM, Med-PaLM-2), Microsoft, and OpenAI (GPT-4) to further responsible advancements in AI.
I have a deep appreciation for open-source work and contribute to projects including Tensorflow, Pytorch Geometric and HuggingFace. In December 2022, I noticed structured output issues in large language models and developed Promptify, which received encouraging feedback on GitHub and assisted relief efforts during the Turkey-Syria earthquake. During COVID-19 pandemic in March 2020, I initiated a project to capture cough and breath sounds via phone to classify COVID-19 coughs using deep learning, with the aim of aiding doctors in rapid pre-screening of patients.
If you are still reading, here is more about me: Apart from my research life, I’m drawn to activities like Boxing, Jiu-Jitsu and Chess. I enjoy spending time in nature, Observation, and Philosophy. I have realized to some extent that everything is connected; there is neither good nor bad; there is neither positive nor negative. I often displace myself away from social noise, take a seat, and try to see, observe, rather than just looking at it. In the process, I naturally learn.
Note: I am looking for a funded PhD opportunity, especially if it fits my Responsible Generative AI, Multimodal LLMs, Geometric Deep Learning, and Healthcare AI skillset.
news
Oct 10, 2023 | Our work on Hallucination Test for Large Language Models was accepted at EMNLP(Conll) 2023. |
---|---|
Jul 29, 2023 | We’re thrilled to announce the release of Promptify 2.0 🎉 - to deal with the structured output issue in LLMs. Promptify is trending on GitHub! ✨ |
Nov 23, 2022 | Our work on Distribution Shift on Question Answering Models was accepted at NeurIPS (Robustness in Sequence Modeling) 2022. |
Oct 1, 2022 | Our work on Federated Learning in Healthcare domain was accepted at ACM 2022. |
Apr 7, 2022 | Our work on Open-domain Question Answering in Medical domain was accepted at Conference on Health, Inference, and Learning (CHIL) 2022. |
Dec 11, 2020 | We worked on ML based discrepancy identification and data reconciliation tool Smart Data Query & Smart Auto Mapper to accelerate COVID vaccine trial. Full details can be found at Pfizer page |